Self-supervised learning method boosts data efficiency by 10x for IMU-based analysis of forces during walking
Collaborators
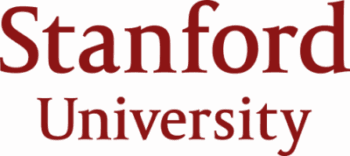
In the field of biomechanics, advancements in deep learning have accelerated the quest for precise human movement assessments. Inertial measurement units (IMUs) — like those used in your smartphone for counting steps — are promising sources of data for such an assessment. However, there’s a catch: IMU-based estimates of a key biomechanical metric, 3-D ground reaction forces (GRFs), are not easily computed. They demand vast amounts of labeled data for deep learning model training, which is not available for most of the daily tasks.
Considering this challenge, a team led by Wu Tsai Human Performance Alliance postdoctoral scholar Tian Tan from Stanford University wondered: Can the power of self-supervised learning (SSL), where algorithms learn from massive datasets without the burden of extensive labeling, produce accurate estimates of GRF from IMUs?
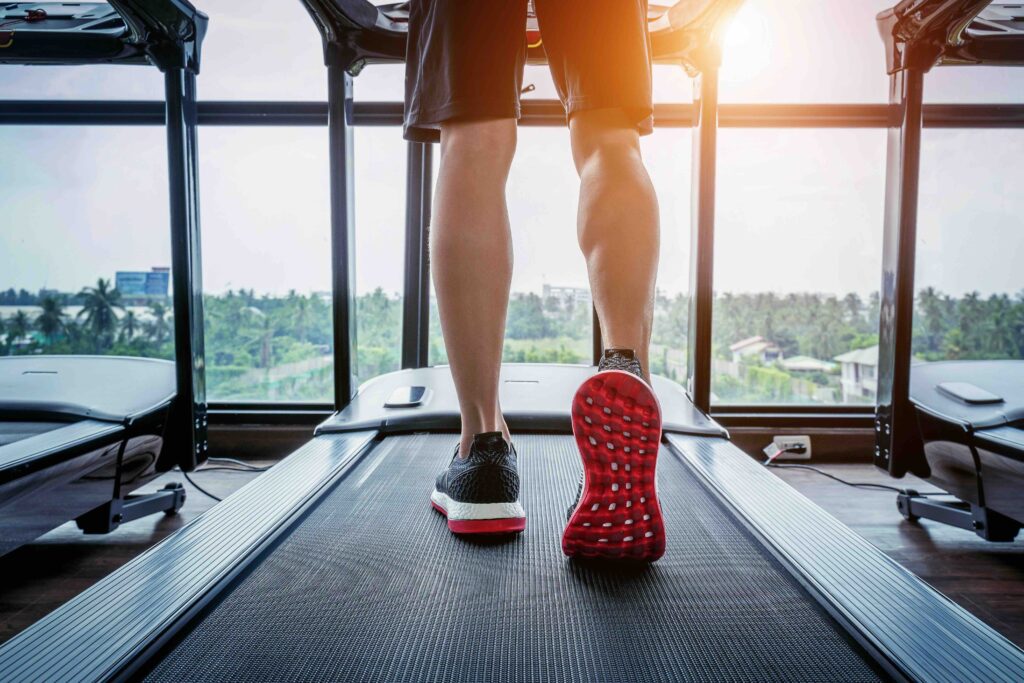
Image credit: Adobe Free Photos/tawatchai1990
Published in IEEE Transactions on Biomedical Engineering, they performed SSL by masking and reconstruction, where a random portion of the IMU data is hidden from the input and used as the output for SSL pre-training. They found that SSL pre-training significantly improved the accuracy of GRF estimation during walking tasks compared to baseline models trained by conventional supervised learning with the same amount of labeled data.
Indeed, with the SSL, Tan only needed to use 10% of overground walking data and could achieve comparable accuracy to the conventional model trained on 100% of the data, indicating a 10x data efficiency improvement. Even better results were observed for GRF estimates from treadmill walking data, where Tan’s approach produced a 100x data efficiency improvement.
The impact of such work suggests that SSL can leverage real and synthetic IMU datasets to increase the data efficiency of GRF estimation, and thus make it more cost-effective. The research also offers a possible solution for populations, such as those with specific physical disabilities, who are not able to finish long data collection sessions in the lab. SSL can potentially allow such groups to benefit from estimating their GRF with minimum data collection.
“We validated that this works for healthy subjects,” said Tan. “We have not yet tested this for other populations to make sure they do predict those values, but it would be exciting if it does.”
The team has made their code and models open-source.
This study is part of the Wu Tsai Human Performance Alliance Digital Athlete moonshot to develop computational models to guide the training and treatment of athletes. Additional authors of this research include Peter B. Shull, Jennifer L Hicks, Scott D. Uhlrich, and Akshay S. Chaudhari.
Latest News
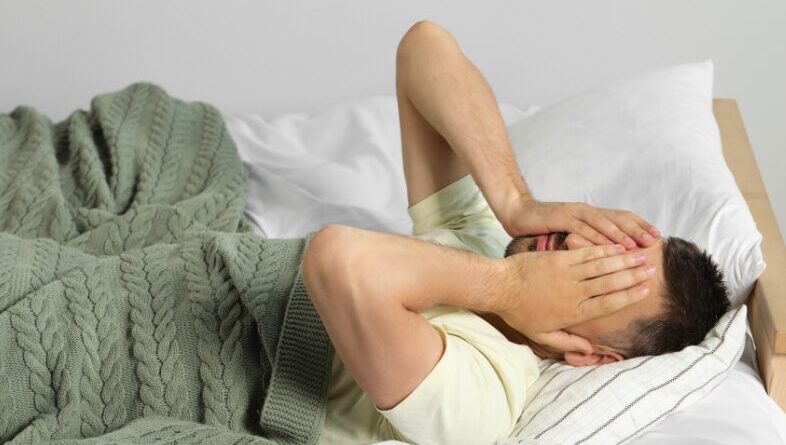
May 29, 2025
Is exercise before sleep linked with poorer sleep?
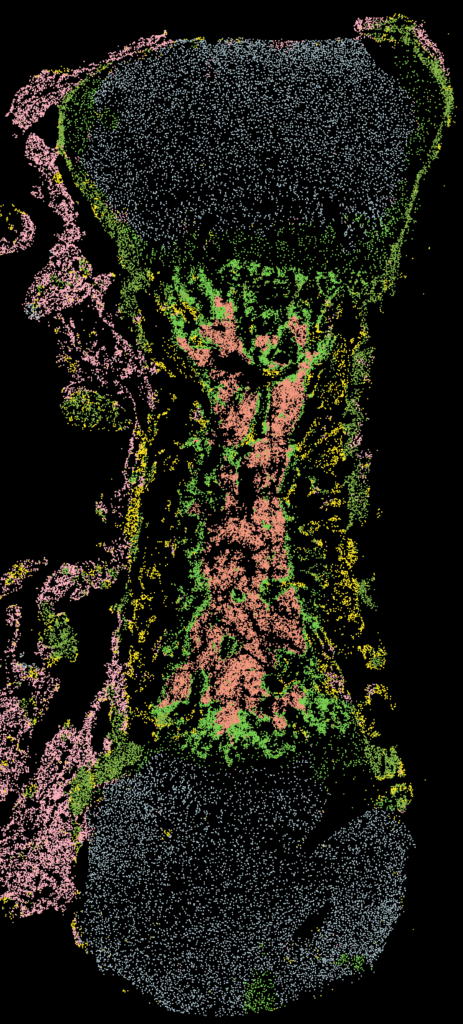
May 23, 2025
Skeletal stem cells key to stronger bones, better healing
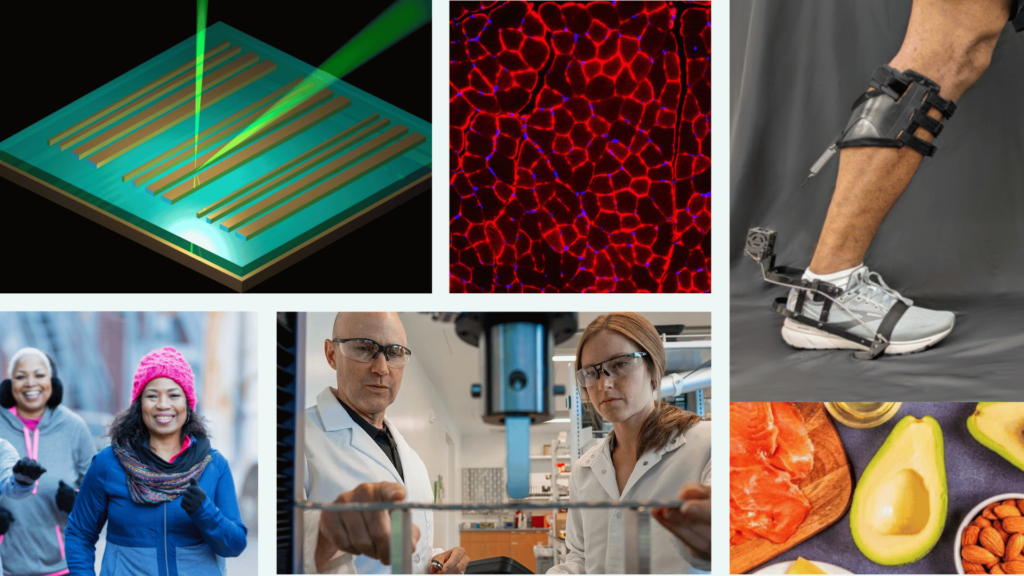
March 13, 2025
Wu Tsai Human Performance Alliance Research Round-Up – March 2025
Get Engaged
Join our mailing list to receive the latest information and updates on the Wu Tsai Human Performance Alliance.