Estimating clinical blood test results with smartwatch data
Collaborators
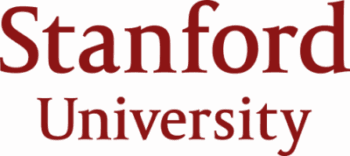
Clinicians and researchers have long been inspired by the potential of consumer-grade wearable sensors, such as smartwatches and smart clothing, to transform clinical care. However, to date, such technology has not resulted in many viable clinical applications – the recent ability to detect atrial fibrillation with smartwatches being a notable exception. Lukasz Kidzinski, a research associate in bioengineering, and colleagues at Stanford University have now developed machine learning models that also correlate smartwatch data with clinical lab tests, such as those that measure the amount of red blood cells.
Their studies elucidate factors that can improve the accuracy of such predictions, such as the use of personalized models versus population-level models. These findings open the door for new clinical uses for smartwatch data.
Michael Snyder, Professor and Chair of Genetics and also a faculty affiliate of the Wu Tsai Human Performance Alliance at Stanford, is the senior author.
Latest News
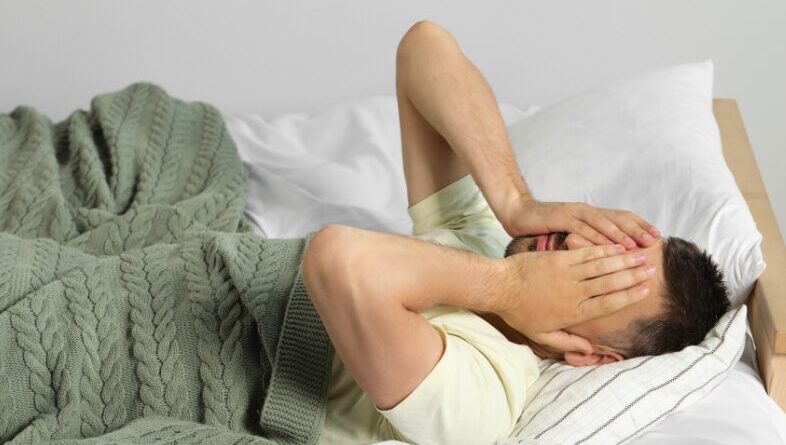
May 29, 2025
Is exercise before sleep linked with poorer sleep?
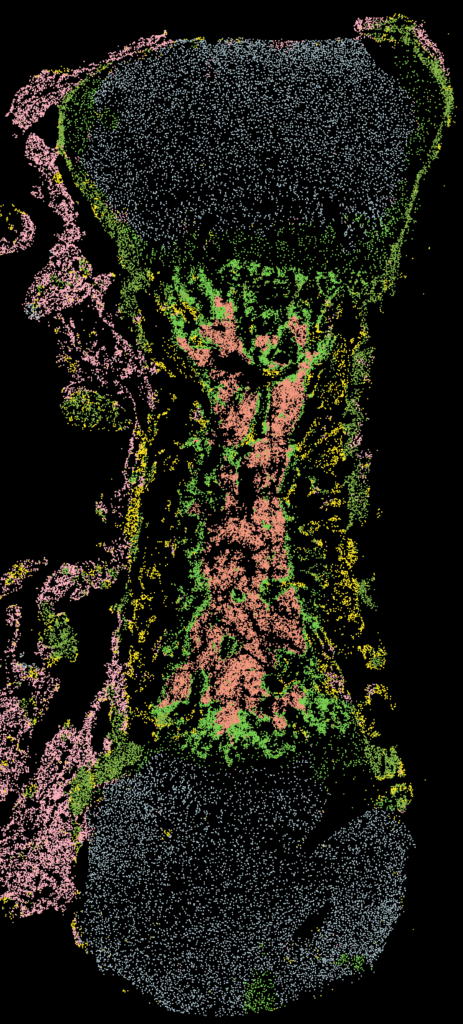
May 23, 2025
Skeletal stem cells key to stronger bones, better healing
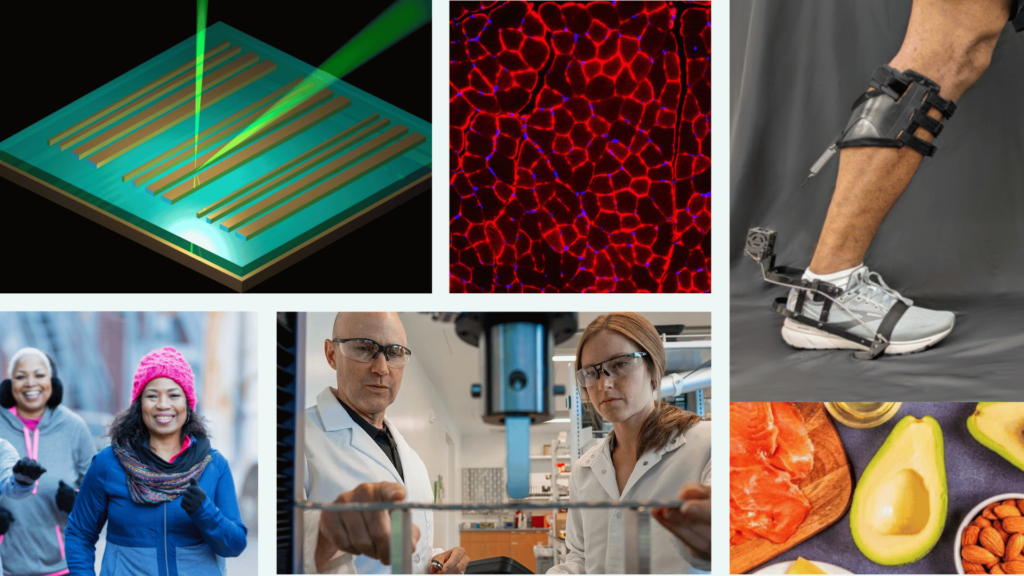
March 13, 2025
Wu Tsai Human Performance Alliance Research Round-Up – March 2025
Get Engaged
Join our mailing list to receive the latest information and updates on the Wu Tsai Human Performance Alliance.